CaLMol: Disentangled Causal Graph LLM for Molecular Relational Learning
Sep 28, 2024·
,,,,,·
0 min read

Peiwen Li
Xin Wang
Zeyang Zhang
Linxin Xiao
Yang Li
Wenwu Zhu
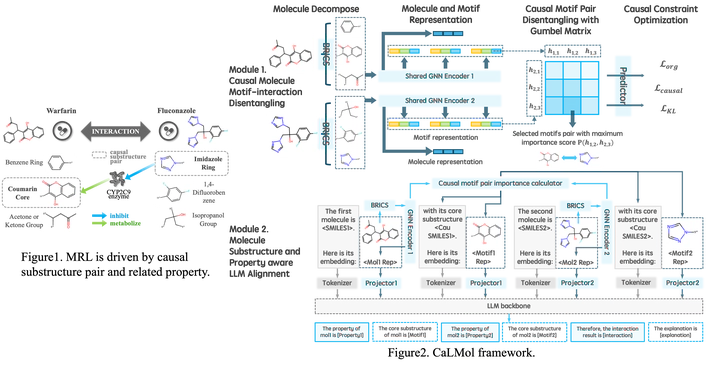
Abstract
Molecular Relational Learning (MRL), focused on understanding interactions between molecular pairs, is essential for drug design by utilizing both structural properties and textual knowledge, such as expert documents. However, most existing MRL methods assume static molecular distributions, meaning the distributions remain consistent across training and testing stages. This assumption may lead to the exploitation of variant correlations between structures and texts regarding interactions, thereby failing in the ubiquitous scenarios involving new drug predictions. To bridge this gap, we investigate zero-shot MRL by leveraging invariant relationships between molecular texts and structures w.r.t interactions for new molecules, which is largely unexplored in the literature and is highly non-trivial with following challenges: 1) How to disentangle molecular structure components between each pair to intrinsically determine interactions and address potential structural distribution shift issues for new drugs? 2) How to align molecular structures with semantic textual information to achieve invariant molecular relation predictions for new drugs? To tackle these challenges, we propose a novel Causally Disentangled Invariant Graph Large Language Model (LLM) for Molecular Relational Learning (CaLMol), capable of exploiting invariant molecular relationships to predict interactions for new drugs. Specifically, we propose Causal Molecule Substructure Disentanglement to capture the invariant well-recognized substructure pair for a specific molecule interaction. Then, we propose Molecule Structure and Property aware LLM Alignment to use molecule (with invariant substructure)-textual property pair to align structure information to semantic information, and use them together to guide the interaction prediction. On this basis, LLM can also provide further explanations. Extensive experiments on qualitative and quantitative tasks including 7 datasets demonstrate that our proposed CaLMol achieves advanced performance on predicting molecule interactions involving new molecules.
Type
Publication
In Submission